Deep Exploration of non-Euclidean Data with Geometric and Topological Representation Learning (22w5125)
Organizers
Guy Wolf (Université de Montréal)
Ladislav Rampasek (Université de Montréal)
Bastian Rieck (Helmholtz Munich)
Ira Ktena (DeepMind - Google)
Description
The Banff International Research Station will host the "Deep Exploration of non-Euclidean Data with Geometric and Topological Representation Learning" workshop at the UBC Okanagan campus in Kelowna, B.C., from July 10 - 15, 2022.
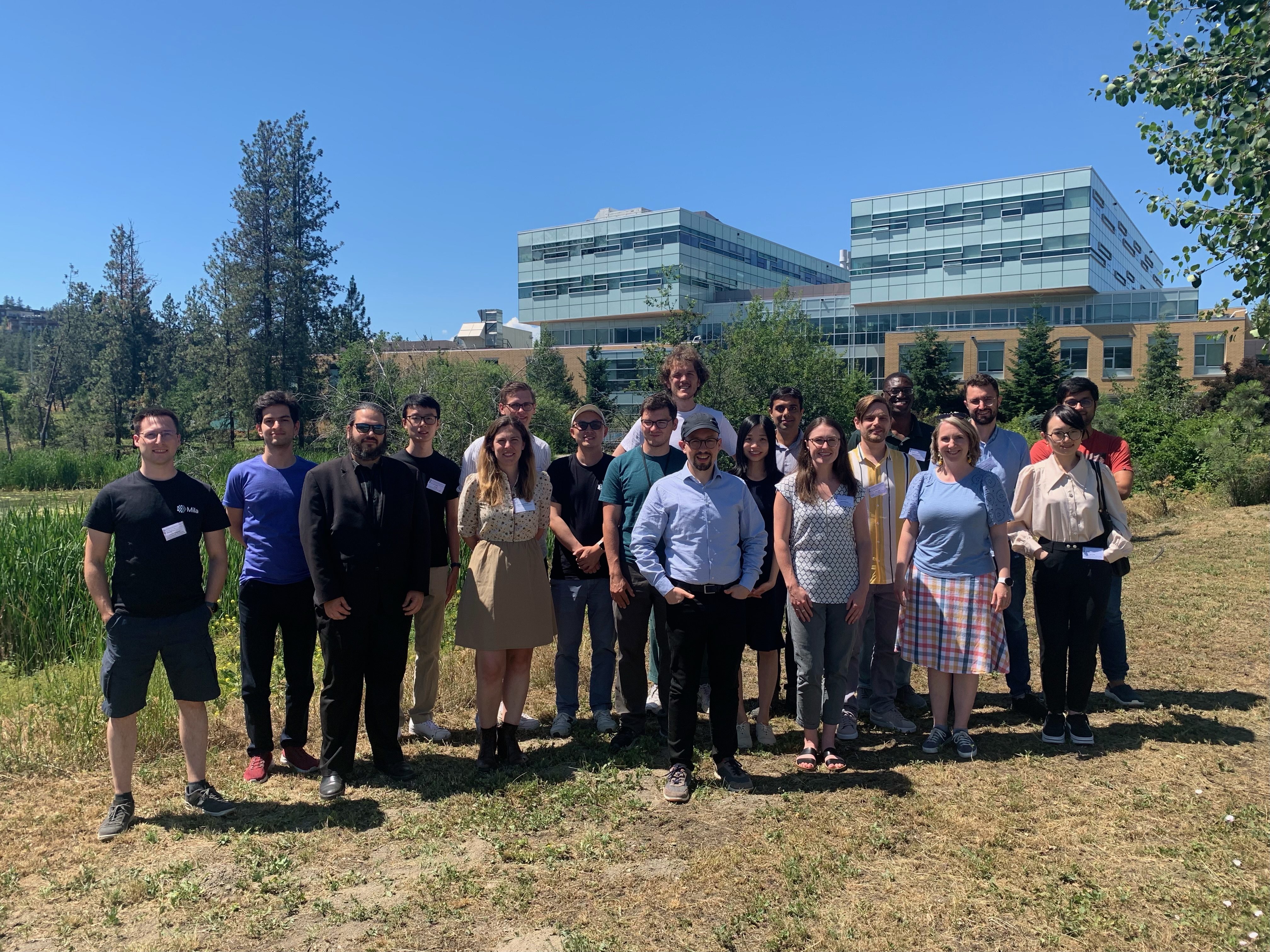
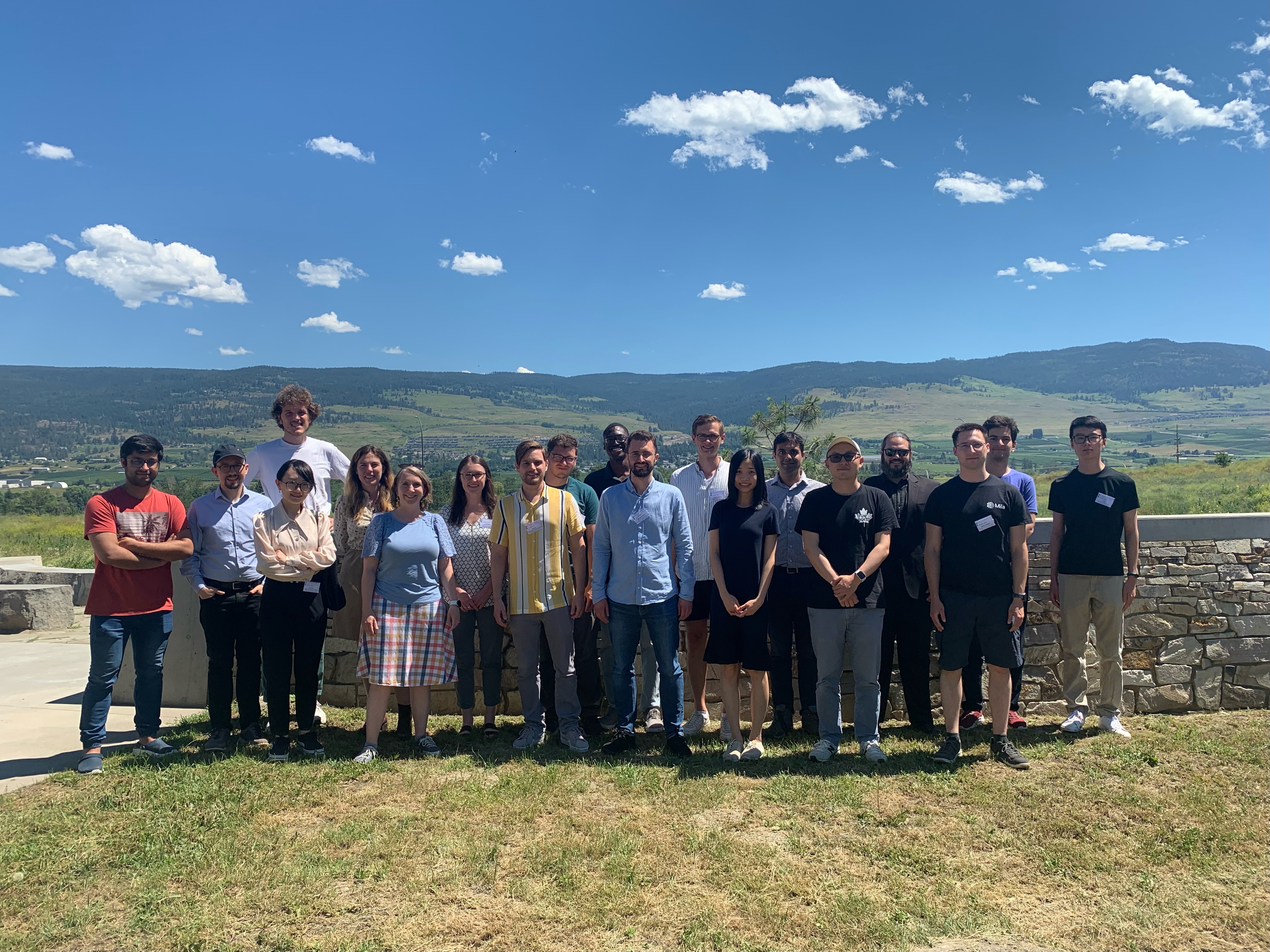
The machine learning revolution of recent years showcased the potential of analyzing massive datasets and using the internal representations that are automatically learned as algorithms are trained to perform (e.g., predictive) tasks on data, which in turn led to major advances in numerous fields of research and technology under the domains of machine learning, artificial intelligence and data science. Along the way, an increasingly evident understanding was formed indicating that most data cannot be modeled via simple Euclidean structures, such as spatiotemporal ones traditionally encountered in images, videos or audio signals. Modern data originating from social networks, biomedical and biomolecular measurements, transportation networks, sensor networks, and numerous other fields often have much more intricate structures, relations, and interactions that can only be captured by decidedly “non-Euclidean” data geometries, as complex and captivating as the famous drawings of M.C. Escher. The challenge of formulating computational tools to describe, infer and leverage such geometries in real-world data analysis and representation learning has been met with numerous developments spread over a wide range of computational fields in mathematics, statistics, computer science, and engineering. However, current progress is spread over various parallel strands of research, each offering separate partial perspectives on the task of formulating robust and meaningful descriptors of intrinsic data geometry.
To fundamentally advance the frontiers of geometric and topological representation learning, a new unified perspective is required, pooling together the wide range of ideas proposed in recent years into an overarching cross-disciplinary body of knowledge. In this workshop, we aim to build the foundations for such development by bringing together leading experts and researchers from multiple disciplines and fields, including manifold learning, graph representation learning, topological data analysis, and graph signal processing. The direct interdisciplinary interaction made possible by this workshop will enable the dissemination of knowledge and a clear view of the current state of the art in participating fields. This, in turn, is expected to result in a distilled long-term vision as well as a short term roadmap for realizing the potential posed by our increasing understanding of intrinsic data geometry and achieving new breakthroughs enabled by it.
The Banff International Research Station for Mathematical Innovation and Discovery (BIRS) is a collaborative Canada-US-Mexico venture that provides an environment for creative interaction as well as the exchange of ideas, knowledge, and methods within the Mathematical Sciences, with related disciplines and with industry. BIRS is supported by Canada's Natural Science and Engineering Research Council (NSERC), the U.S. National Science Foundation (NSF), Alberta's Advanced Education and Technology, and Mexico's Consejo Nacional de Ciencia y Tecnología (CONACYT).
Travel details:
The Kelowna campus is best reached by airplane. See here for detailed information about reaching the campus. We recommend booking flights early on, with a potential eye towards additional conferences that happen in the summer of 2022, such as ICML.